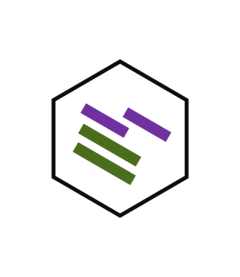
Data_processing
Arvind Iyer
2025-04-04
data_processing.Rmd
- The goal of SelectSim pacakge is to implement the SelectSim methodology to infer inter-dependencies between functional alterations in cancer.
-
functional
package provides function to generate the backgorund model and other utilites functions.
Installation
- You can install the development version of SelectSim from GitHub with:
# install.packages("devtools")
devtools::install_github("CSOgroup/SelectSim",dependencies = TRUE, build_vignettes = TRUE)
- For more details on installation refer to INSTALLATION
Example
We will process LUAD MAF dataset from TCGA provided with the package.
We will also use oncokb v3.9 cancer genes and mutations to filter the maf to create the gam provided with the pacakge.
NOTE: This an example to prcoess the MAF file. To know more about how we prcossed the all MAF file to run_data object, please refer to SelectSim_analysis repository.
library(SelectSim)
library(dplyr)
#>
#> Attaching package: 'dplyr'
#> The following objects are masked from 'package:stats':
#>
#> filter, lag
#> The following objects are masked from 'package:base':
#>
#> intersect, setdiff, setequal, union
library(tictoc)
## Load the data provided with the package
data(luad_maf, package = "SelectSim")
data(oncokb_genes, package = "SelectSim")
data(oncokb_truncating_genes, package = "SelectSim")
data(variant_catalogue, package = "SelectSim")
# Check the MAF
dim(luad_maf)
#> [1] 220734 8
- Let print number of lines and number of samples
input_maf <- luad_maf
print(paste('##### Number of lines ####',nrow(input_maf),sep="->"))
#> [1] "##### Number of lines ####->220734"
genes_to_consider = oncokb_genes
print(paste('##### Number of genes ####',length(genes_to_consider),sep="->"))
#> [1] "##### Number of genes ####->396"
- Let create a table schema of mutations to conisder and columns defined in maf file
mutation_type = list(
'ignore' = c("Silent","Intron","RNA","3'UTR","5'UTR","5'Flank","3'Flank","IGR"),
'truncating'= c('Frame_Shift_Del','Frame_Shift_Ins','In_Frame_Del','In_Frame_Ins','Nonsense_Mutation','Nonstop_Mutation','Splice_Region','Splice_Site','Translation_Start_Site'),
'missense' = c('Missense_Mutation')
)
custom_maf_schema = list(
'name' = 'custom_maf',
'column' = list(
'gene' = 'Hugo_Symbol'
, 'gene.name' = 'Hugo_Symbol'
, 'sample' = 'sample'
, 'sample.name' = 'sample'
, 'mutation.type' = 'Variant_Classification'
, 'mutation' = 'HGVSp_Short'
),
'mutation.type' = mutation_type
)
- Number of samples in the maf file
mut_samples = unique(input_maf[, custom_maf_schema$column$sample])
print(paste('##### Number of samples ####',length(mut_samples),sep="->"))
#> [1] "##### Number of samples ####->502"
- Filter the maf file to include oncokb cancer genes
maf_genes = filter_maf_gene.name(input_maf, genes = genes_to_consider, gene.col = custom_maf_schema$column$gene)
print(paste('##### Number of lines ####',nrow(maf_genes),sep="->"))
#> [1] "##### Number of lines ####->6708"
Genrating the GAMs
- Let generate the truncating data
- We generate the GAM consider the genes to consider truncating mutations.
- We also create a TMB dataframe which takes all truncating mutation of all genes in consideration.
# Creating Truncating GAM
tic('##### Creating Truncating GAM ####')
maf_trunc = filter_maf_truncating(maf_genes,genes=oncokb_truncating_genes, custom_maf_schema)
input_maf_trunc<-filter_maf_truncating(input_maf, custom_maf_schema)
truncating_tmb <- data.frame('sample'=mut_samples,'mutation'=rep(0,length(mut_samples)))
rownames(truncating_tmb)<-mut_samples
temp <- input_maf_trunc %>% count(sample)
rownames(temp)<-temp$sample
truncating_tmb[intersect(truncating_tmb$sample,temp$sample),]$mutation <-temp[intersect(truncating_tmb$sample,temp$sample),'n']
tcga_truc_gam = maf2gam(maf_trunc,
sample.col = custom_maf_schema$column$sample,
gene.col = custom_maf_schema$column$gene,
value.var = 'Variant_Classification',
samples = mut_samples,
genes = genes_to_consider,
fun.aggregate = length,
binarize=TRUE,
fill=0)
truncating_data <- list('gam'=tcga_truc_gam,
'tmb'=truncating_tmb)
toc()
#> ##### Creating Truncating GAM ####: 0.228 sec elapsed
- Let generate the Missense data
- We generate the GAM with genes and hotspot mutations from oncokb v3.9.
- We also create a TMB dataframe which takes all Missense mutation of all genes in consideration.
# Creating Missense GAM
tic('##### Creating Missense GAM ####')
maf_valid = filter_maf_schema(input_maf,
schema = custom_maf_schema,
column = 'mutation.type',
values = custom_maf_schema[['mutation.type']][['ignore']],
inclusive = FALSE)
missense_maf<-filter_maf_mutation.type(input_maf,
variants = 'Missense_Mutation',
variant.col = custom_maf_schema$column$mutation.type)
missense_tmb <- data.frame('sample'=mut_samples,'mutation'=rep(0,length(mut_samples)))
rownames(missense_tmb)<-mut_samples
temp <- missense_maf %>% count(sample)
rownames(temp)<-temp$sample
missense_tmb[intersect(missense_tmb$sample,temp$sample),]$mutation <-temp[intersect(missense_tmb$sample,temp$sample),'n']
t_m = substr(maf_valid[[custom_maf_schema$column$mutation]],3,1000)
t_m1 = gsub('[A-Z]*$', '', t_m)
maf_valid$HGVSp_Short_fixed = t_m1
maf_hotspot = filter_maf_mutations(maf_valid,
variant_catalogue,
maf.col = c(custom_maf_schema$column$gene, 'HGVSp_Short_fixed'),
values.col = c('gene', 'mut'))
missense_tcga_gam = maf2gam(maf_hotspot,
sample.col = custom_maf_schema$column$sample,
gene.col = custom_maf_schema$column$gene,
value.var = 'Variant_Classification',
samples = mut_samples,
genes = genes_to_consider,
fun.aggregate = length,
binarize=TRUE,
fill=0)
missesne_data <- list('gam'=missense_tcga_gam,
'tmb'=missense_tmb)
toc()
#> ##### Creating Missense GAM ####: 1.873 sec elapsed
Genrating the run_object to run SelectX
- We create a run_object data which is list object which consists of
- M: a list object of GAMs which is presence absence matrix of alterations
- tmb: a list object of tumor mutation burden as data frame with column names (should be) as sample and mutationn
- sample.class a named vector of sample annotations
- alteration.class a named vector of alteration annotations
gene_to_take <- colnames(missesne_data$gam)
order <- rownames(missesne_data$gam)
data <-list('M'=list('missense'=t(missesne_data$gam[order,gene_to_take]),
'truncating'=t(truncating_data$gam[rownames(missesne_data$gam[order,]),gene_to_take])),
'tmb'=list('missense'=missesne_data$tmb[order,],
'truncating'=truncating_data$tmb[order,]))
alteration_covariates <- rep('MUT',ncol(missesne_data$gam[order,gene_to_take]))
names(alteration_covariates)<-colnames(missesne_data$gam[order,gene_to_take])
sample_covariates<-rep('LUAD',length(order))
names(sample_covariates)<-order
run_data <- list('M'=data,'sample.class' = sample_covariates,'alteration.class' = alteration_covariates)
str(run_data)
#> List of 3
#> $ M :List of 2
#> ..$ M :List of 2
#> .. ..$ missense : num [1:396, 1:502] 0 0 0 0 0 0 0 0 0 0 ...
#> .. .. ..- attr(*, "dimnames")=List of 2
#> .. .. .. ..$ : chr [1:396] "AKT1" "ATM" "BRAF" "CDKN2A" ...
#> .. .. .. ..$ : chr [1:502] "TCGA-05-4244-01" "TCGA-05-4249-01" "TCGA-05-4250-01" "TCGA-05-4382-01" ...
#> .. ..$ truncating: num [1:396, 1:502] 0 0 0 0 0 0 0 0 0 0 ...
#> .. .. ..- attr(*, "dimnames")=List of 2
#> .. .. .. ..$ : chr [1:396] "AKT1" "ATM" "BRAF" "CDKN2A" ...
#> .. .. .. ..$ : chr [1:502] "TCGA-05-4244-01" "TCGA-05-4249-01" "TCGA-05-4250-01" "TCGA-05-4382-01" ...
#> ..$ tmb:List of 2
#> .. ..$ missense :'data.frame': 502 obs. of 2 variables:
#> .. .. ..$ sample : chr [1:502] "TCGA-05-4244-01" "TCGA-05-4249-01" "TCGA-05-4250-01" "TCGA-05-4382-01" ...
#> .. .. ..$ mutation: num [1:502] 163 253 270 1328 100 ...
#> .. ..$ truncating:'data.frame': 502 obs. of 2 variables:
#> .. .. ..$ sample : chr [1:502] "TCGA-05-4244-01" "TCGA-05-4249-01" "TCGA-05-4250-01" "TCGA-05-4382-01" ...
#> .. .. ..$ mutation: num [1:502] 24 45 40 206 17 18 73 31 176 108 ...
#> $ sample.class : Named chr [1:502] "LUAD" "LUAD" "LUAD" "LUAD" ...
#> ..- attr(*, "names")= chr [1:502] "TCGA-05-4244-01" "TCGA-05-4249-01" "TCGA-05-4250-01" "TCGA-05-4382-01" ...
#> $ alteration.class: Named chr [1:396] "MUT" "MUT" "MUT" "MUT" ...
#> ..- attr(*, "names")= chr [1:396] "AKT1" "ATM" "BRAF" "CDKN2A" ...
- Save the
run_data
and check the introduction vignette to see how to run selectX to discover EDs.
SessionInfo
# Print the sessionInfo
sessionInfo()
#> R version 4.4.2 (2024-10-31)
#> Platform: x86_64-conda-linux-gnu
#> Running under: AlmaLinux 9.3 (Shamrock Pampas Cat)
#>
#> Matrix products: default
#> BLAS/LAPACK: /mnt/ndata/arvind/envs/selectsim_R/lib/libopenblasp-r0.3.29.so; LAPACK version 3.12.0
#>
#> locale:
#> [1] LC_CTYPE=en_US.UTF-8 LC_NUMERIC=C
#> [3] LC_TIME=en_US.UTF-8 LC_COLLATE=en_US.UTF-8
#> [5] LC_MONETARY=en_US.UTF-8 LC_MESSAGES=en_US.UTF-8
#> [7] LC_PAPER=en_US.UTF-8 LC_NAME=C
#> [9] LC_ADDRESS=C LC_TELEPHONE=C
#> [11] LC_MEASUREMENT=en_US.UTF-8 LC_IDENTIFICATION=C
#>
#> time zone: Europe/Zurich
#> tzcode source: system (glibc)
#>
#> attached base packages:
#> [1] stats graphics grDevices utils datasets methods base
#>
#> other attached packages:
#> [1] tictoc_1.2.1 dplyr_1.1.4 SelectSim_0.0.1.5
#>
#> loaded via a namespace (and not attached):
#> [1] sass_0.4.9 generics_0.1.3 tidyr_1.3.1 rstatix_0.7.2
#> [5] stringi_1.8.7 lattice_0.22-6 digest_0.6.37 magrittr_2.0.3
#> [9] evaluate_1.0.3 grid_4.4.2 iterators_1.0.14 fastmap_1.2.0
#> [13] plyr_1.8.9 Matrix_1.7-3 foreach_1.5.2 doParallel_1.0.17
#> [17] jsonlite_2.0.0 backports_1.5.0 Formula_1.2-5 purrr_1.0.4
#> [21] doRNG_1.8.6.2 scales_1.3.0 codetools_0.2-20 textshaping_0.4.0
#> [25] jquerylib_0.1.4 abind_1.4-5 cli_3.6.4 rlang_1.1.5
#> [29] munsell_0.5.1 cachem_1.1.0 yaml_2.3.10 tools_4.4.2
#> [33] parallel_4.4.2 reshape2_1.4.4 ggsignif_0.6.4 colorspace_2.1-1
#> [37] ggplot2_3.5.1 ggpubr_0.6.0 rngtools_1.5.2 RcppZiggurat_0.1.8
#> [41] Rfast_2.1.0 broom_1.0.8 vctrs_0.6.5 R6_2.6.1
#> [45] ggridges_0.5.6 lifecycle_1.0.4 stringr_1.5.1 fs_1.6.5
#> [49] car_3.1-3 htmlwidgets_1.6.4 ragg_1.3.3 pkgconfig_2.0.3
#> [53] desc_1.4.3 RcppParallel_5.1.9 pkgdown_2.1.1 pillar_1.10.1
#> [57] bslib_0.9.0 gtable_0.3.6 Rcpp_1.0.14 glue_1.8.0
#> [61] systemfonts_1.2.1 xfun_0.51 tibble_3.2.1 tidyselect_1.2.1
#> [65] knitr_1.50 htmltools_0.5.8.1 rmarkdown_2.29 carData_3.0-5
#> [69] compiler_4.4.2